New research problem validity paper (open access in Perspectives on Psychological Science)
My coauthors Stefan Thau (INSEAD), Madan Pillutla (Indian School of Business) and I wrote a new paper called"Research Problem Validity in Primary Research: Precision and Transparency in Characterizing Past Knowledge". The article identifies a major issue in the field of psychological science: the lack of precision and transparency in the characterization of past research that feeds into problem statements in primary research articles. The article is published open access in Perspectives on Psychological Science (Schweinsberg et al., 2023).
Four types of validity (Cook & Campbell, 1979, Chapter 2; Shadish et al., 2002, Chapters 2-3) are typically discussed to evaluate the study design and data analysis steps of the research process. Validity standards are not necessary for the idea generation step of research which may include personal insights, creative discoveries, or any other way to generate an initial idea.
However, new validity standards are needed for the step that is currently a black box in the research process: the review of past research and thesubsequent identification of research problems (see Figure 1).
We therefore do not try to improve existing validity types, but instead propose a new type of validity: research problem validity. Research problem validity seeks to define, scrutinize, and improve the extent of correctness of research problems in primary research.
Research problem validity helps scholars identify the right research problem, whereas other types of validity help solve this problem in the right way.
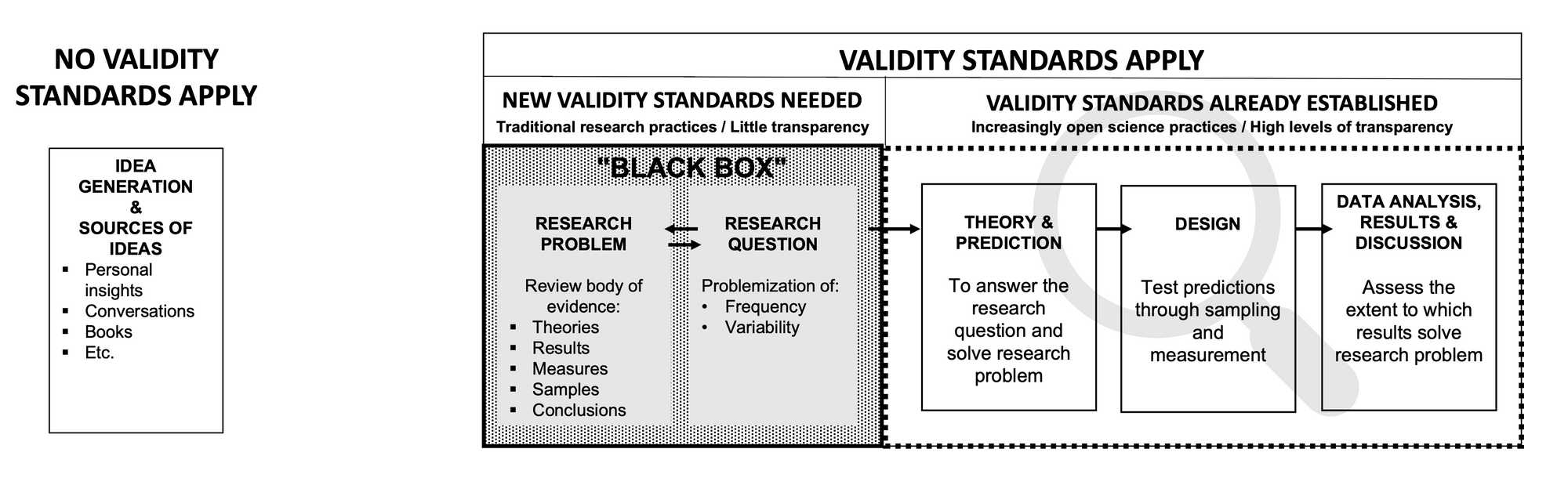
Research Problem Validity Supersedes Other Types of Validity
Research problems determine research questions,and therefore direct many decisions of the subsequent research process (Kerlinger, 1986). The four validity types that are commonly used and taught in method courses therefore become meaningful after research problem validity has been established. The construction of research problems and establishing their validity can be conceptualized as a superordinate step to development of theory and predictions, just as theory and prediction can be thought of as superordinate steps to research design and statistical analyses (c.f. Fiedler et al., 2021).
Definition of Research Problem Validity
Identifying a research problem in primary research consists of a review of past research that reveals two or more factors that bring about a contradiction or undesirable consequence (Clark et al., 1977, p. 6; Kerlinger, 1986, p. 17; Pillutla & Thau, 2013).
The key to identifying research problems is a review of past research. This review involves making quantitative judgments about features of past research with regards to the frequency (e.g., “most,” “few”) and/or variability (e.g., “on the one hand, on the other hand”) of theories, measures, samples, tasks, analyses, results, or conclusions.
These features are then problematized by making apparent one or multiple contradictions with other scientific knowledge and by pointing out the undesirability of not having an answer to these contradictions. The problematized review of previous work is a crucial step that directly determines the research question and the professed magnitude of the potential contribution of the research. It informstheory, predictions, and all other subsequent aspects of the research process, including design and data analysis (Hernon & Schwartz, 2007).
Our definition of research problem validity highlights the degree of truthfulness of an inference as central to evaluating validity. More specifically, by truthful we mean the extent to which the judgment is correct given the available evidence in past research. Multiple criteria need to be considered to establish validity (Cook & Campbell, 1979, Chapter 2; Shadish et al., 2002, Chapters 2-3). The two key criteria to evaluate research problem validity are the extent to which the judgment is precise, and how transparent it is how judgment came about.
Precision in Reviews of Past Research
By precision, we refer to the degree to which an inference is exact and accurately judges the available research. A judgment such as “most studies” is vague, because it could refer to anything between 50.01% and 99.99% of all studies (Partee, 1989; Rett, 2018). Instead, a more precise and informative inference could say that “70% of reviewed studies” show a certain characteristic. We argue that imprecise summary statements in primary research could bias the interpretation of quantitative judgments.
Vague summaries about past research could create collective misunderstandings as it is unclear what type of summary the body of evidence warrants, creating a knowledge gap.
Transparency Enables Precision in Reviews of Past Research
Transparency describes how clearly the authors communicate how they came toconclusions about past research. Transparency also describes the extent to which authors were open and explicit about the processes and methods they used to search, code, and characterize past research (Denyer & Tranfield, 2009). Transparency enables precision because it provides both an incentive to be more precise and to be more accurate. When researchers describe how their assessment of “most studies” came about, transparency incentiviezes them to show which sample of studies they reviewed, how these studies were coded, and what this implies for certain features of past work. Therefore, transparencyenables precision.
Summary of our paper (Abstract):
Four types of validity evaluate the approximate truth of inferences communicated by primary research. However, current validity frameworks ignore the truthfulness of empirical inferences that are central to research problem statements. Problem statements contrast a review of past research with other knowledge that extend, contradict, or call into question specific features of past research. Authors communicate empirical inferences, or quantitative judgments about the frequency (e.g., “few,” “most”) and variability (e.g., “on the one hand, on the other hand”) in their reviews of existing theories, measures, samples, or results. We code a random sample of primary research articles and show that 83% of quantitative judgments in our sample are both vague and their origin non-transparent, making it difficult to assess their validity. We review validity threats of current practices. We propose that documenting the literature search, how the search was coded, along with quantification facilitates more precise judgments and makes their origin transparent. This practice enables research questions that are more closely tied to the existing body of knowledge and allows for more informed evaluations of the contribution of primary research articles, their design choices, and how they advance knowledge. We discuss potential limitations of our proposed framework.
References:
Clark, D., Guba, E., & Smith, G. (1977). Functions and definitions of functions of a research proposal. Bloomington: College of Education Indiana University.
Cook, T. D., & Campbell, D. T. (1979). Quasi-experimentation: Design & analysis for field settings.
Denyer, D., & Tranfield, D. (2009). Producing a systematic review. In D. A. Buchanan & A. Bryman (Eds.), The Sage handbook of organizational research methods (pp. 671-689). Sage Publications.
Fiedler, K., McCaughey, L., & Prager, J. (2021). Quo vadis, methodology? The key role of manipulation checks for validity control and quality of science. Perspectives on Psychological Science, 16(4), 816-826. https://doi.org/10.1177/1745691620970602
Hernon, P., & Schwartz, C. (2007). What is a problem statement? Library & Information Science Research, 29(3), 307-309. https://doi.org/10.1016/j.lisr.2007.06.001
Kerlinger, F. N. (1986). Foundations of behavioral research (3rd ed.). Harcourt Brace Jovanovich College Publishers.
Partee, B. H. (1989). Many quantifiers. ESCOL 89: Proceedings of the Eastern States Conference on Linguistics, Columbus, OH.
Pillutla, M. M., & Thau, S. (2013). Organizational sciences’ obsession with “that’s interesting!”. Organizational Psychology Review, 3(2), 187-194. https://doi.org/10.1177/2041386613479963
Rett, J. (2018). The semantics of many, much, few, and little. Language and Linguistics Compass, 12(1), e12269. https://doi.org/https://doi.org/10.1111/lnc3.12269
Schweinsberg, M., Thau, S., & Pillutla, M. M. (2023). Research problem validity in primary research: Precision and transparency in characterizing past knowledge. Perspectives on Psychological Science, 18, 1230-1243. https://doi.org/https://doi.org/10.1177/17456916221144990
Shadish, W. R., Cook, T. D., & Campbell, D. T. (2002). Experimental and quasi-experimental designs for generalized causal inference. Houghton Mifflin Company.